RESEARCH TRACK
Valuable and Responsible AI Systems
In the Summit research track, EAISI researchers and partners will present their latest findings, specifically around the theme of the real power of AI. In the midst of AI developments that lead to doubts or even fear, we put some necessary focus on the positive influence AI has and will have on our lives and the real world around us. Please join the discussions and get to know in detail what is happening in the center of Brainport’s AI research.
At EAISI 900+ AI-researchers do research on AI systems where the physical, digital, and human worlds come together. EAISI aims to get to a better understanding, better designs, better models, and better decisions in the application areas of Health, Mobility, and High-Tech Systems.
TIMETABLE
11:30 Duo presentation | Zaharah Bukhsh & Kasper Hendriks
12:00 Pitches | Patricia Kahr, Andrii Kompanets & Apoorva Singh
12:20 Presentation | Fons van der Sommen
12:40 Presentation | Carlos Zednik
13:00 Lunch break & Expo
14:20 Presentation | Remco Duits
14:40 Duo presentation | Josette Gevers & Travis Wiltshire
15:05 Duo presentation | Aaqib Saeed & Hareld Kemps
15:30 Presentation | Jakub Tomczak
15:50 Triple Presentation | Elena Torta, Marco Alonso & Koen de Vos
16:15 Central closing | with mayor Jeroen Dijsselbloem
16:30 Expo, networking & drinks
18:00 End Time
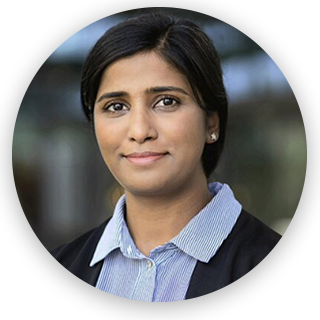
Zaharah Bukhsh (duo talk)
Assistant professor at Eindhoven University of Technology
Decision-making with deep reinforcement learning
Deep learning has revolutionized data-driven models, enabling the mastery of complex tasks by learning from vast amounts of (labeled) data.
View profile on TU/e website
Abstract
Deep reinforcement learning (RL) takes this progress a step further by enabling decision-making under uncertainty, where the goal is not only to perceive and classify but also to take actions and consider their long-term consequences.
In this talk, we will present real-world applications of deep RL in diverse domains, from maintenance planning of underground utilities and quay walls to the collaborative order picking problem in Vanderlande warehouses. We will show how deep RL empower intelligent decision-making in complex and dynamic environments.
The talk will conclude by providing valuable lessons learned from industry expert, shedding light on the challenges and opportunities that arise when applying deep RL in real-world settings.
Kasper Hendriks (duo talk)
Innovation engineer at Vanderlande
Decision-making with deep reinforcement learning
Deep learning has revolutionized data-driven models, enabling the mastery of complex tasks by learning from vast amounts of (labeled) data.
View profile on LinkedIn
Abstract
Deep reinforcement learning (RL) takes this progress a step further by enabling decision-making under uncertainty, where the goal is not only to perceive and classify but also to take actions and consider their long-term consequences.
In this talk, we will present real-world applications of deep RL in diverse domains, from maintenance planning of underground utilities and quay walls to the collaborative order picking problem in Vanderlande warehouses. We will show how deep RL empower intelligent decision-making in complex and dynamic environments.
The talk will conclude by providing valuable lessons learned from industry expert, shedding light on the challenges and opportunities that arise when applying deep RL in real-world settings.
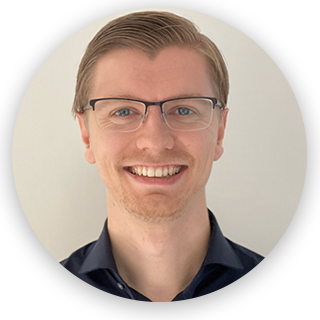
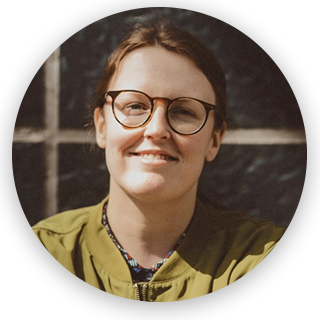
Patricia Kahr
PhD Candidate in the Human-Technology Interaction group.
Performance is Not Everything! Learning What Promotes Trustworthy Human-AI Collaborations. Insights from a Case Study in Logistics Planning
An important area of research on human-AI interaction focuses on promoting trust and reliance in AI decision support systems.
View profile on TU/e website
Abstract
Research in this area is thriving, and it is evident that trust and reliance in AI systems are often not well-calibrated for users: experts frequently find it challenging to depend on the advice from such systems or may outright refuse to use their support for decision-making.
To gain a deeper understanding of real-life collaborations, we conducted semi-structured in-depth interviews with logistics planning experts to explore their experiences with their automated planning system.
Our study sheds light on the overall trust dynamics in this area and the conditions under which experts rely on or deviate from AI recommendations. We highlight the importance of incorporating human needs and special circumstances into AI systems to foster more effective and trustworthy collaboration between humans and AI.
Andrii Kompanets
PhD Candidate at the Steel Structures group at TU/e
AI-aided visual inspection of steel bridges
Automating the current bridge visual inspection practices using drones and image processing techniques is a prominent way to make these inspections more effective, robust, and less expensive.
View profile on TU/e website
Abstract
In our work, we investigate the development of a novel deep-learning method for the detection of fatigue cracks in high-resolution images of steel bridges.
First, we developed a semi-automatic tool based on a geometric tracking algorithm, which allows a fast and accurate pixels-wise annotation of cracks in images. We used the developed tool to generate a challenging benchmark dataset. Further, we conducted series of experiments on this dataset, aiming at the development of a robust, fully-automatic deep-learning method for crack segmentation.
Based on these experiments, multiple discoveries were made and novel solutions were proposed, which helped to significantly increase reliability of crack segmentation system.
To prove the concept of automatic inspection of bridges, we also developed a method to compare our automatic crack segmentation system with the performances of human inspectors.
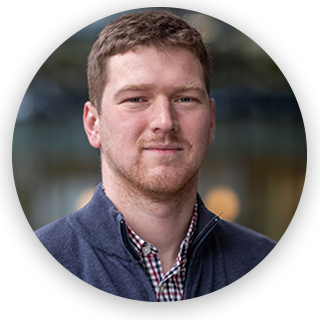
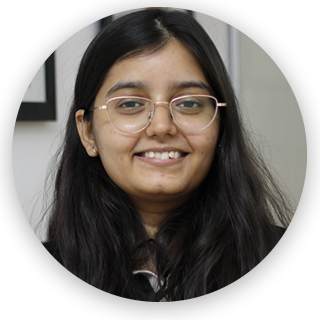
Apoorva Singh
Predictive modeling of large-scale pedestrian dynamics using AI
The current research work, supported by the EAISI EMDAIR project “AICrowd”, aims at quantitatively modelling the dynamics of pedestrian crowds, using tools from AI and System Identification.
View profile on TU/e website
Abstract
It's part of a decade-long endeavor towards establishing a physical understanding and predictive modeling capabilities of crowd flows: an effort at the interface of physics, AI, and engineering.
This understanding is crucial for effective crowd management and designing safe and comfortable urban infrastructure.
Fons van der Sommen
Associate Professor Video Coding & Architectures, TU/e
Robust, self-critical AI for oncology
While the capabilities of modern AI systems keep surpassing expectations for a wide range of applications, their development has mostly driven by their accuracy on standardized data sets.
View profile on TU/e website
Abstract
This empirical approach to model development introduces blind spots towards their application, as evidenced by an increasing number of reports on unstable model behaviour, under minor changes in the unput data.
For healthcare applications, these observations are especially concerning, as unreliable AI output may lead to incorrect diagnoses and treatment.
In this talk, dr. van der Sommen will elaborate on the current lack of model robustness for oncology applications and will discuss methods that could help mitigate this problem.
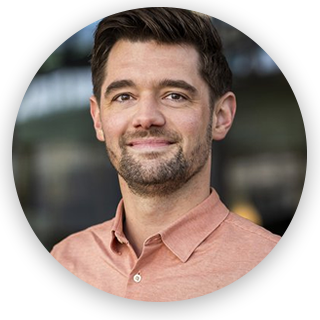
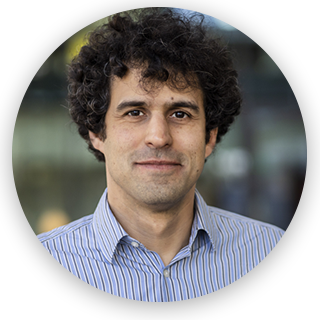
Carlos Zednik
Assistant Professor, TU/e
Cognitive models to understand knowledge-representation in large language models
Harnessing the power of large language models while retaining control over their behavior is one of the central challenges of contemporary artificial intelligence.
View profile on TU/e website
Abstract
One way to meet this challenge is to identify and characterize the knowledge these models possess, or the beliefs that drive their behavior.
In this talk, I present an explanatory strategy that combines methodological principles from cognitive science and explainable AI to characterize the knowledge or beliefs that are represented in state-of-the-art transformer architectures.
This strategy centers on cognitive models, which provide not only predictive power over a particular system's behavior, but which also support systematic and targeted interventions on that behavior.
Remco Duits
Head of the Geometric Learning and Differential Geometry group, Cluster: CASA. Department: Mathematics and Computer Science. EAISI, TU/e.
New Geometric Learning for Medical and Industrial Image Analysis
Our geometrically interpretable networks achieve better classification results in image processing with both less training data and less network complexity.
View profile on TU/e website
Abstract
We show that our geometric image processing is more accurate for various medical and industrial image analysis problems, e.g.: 1) segmentation and tracking of complex vasculature, 2) wall-shear stress estimation of vasculature, 3) segmentation of chips, and 4) crack detection in steel bridges.
To get to state-of-the-art solutions for practical medical and industrial image analysis problems, we develop PDE-based Group Convolutional Neural Networks (PDE-G-CNNs).
In PDE-G-CNNs a network layer is a set of solvers of PDEs (Partial Differential Equations). The PDEs are defined on the space of positions and orientations and provide a geometric design of the roto-translation equivariant neural network.
The network consists of morphological convolutions with kernels solving nonlinear PDEs (HJB equations for max-pooling over Riemannian balls), and linear convolutions solving linear PDEs (convection, fractional diffusion). Common mystifying (ReLU) nonlinearities are now obsolete and excluded.
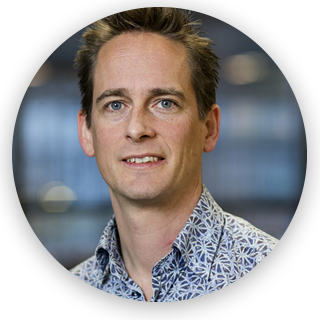
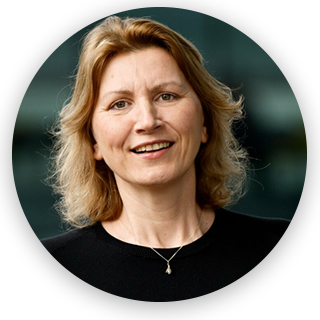
Josette Gevers (duo talk)
Full Professor TU/e
An AI-based feedback system for team support during crisis events
Wearable technology offers a groundbreaking opportunity to provide teams with real-time feedback to enhance their effectiveness in high-stakes crisis environments, such as medical emergencies.
View profile on TU/e website
Abstract
While current wearable technology primarily targets individual users, advancements in AI are unveiling new potentials for capturing and supporting team dynamics in circumstances where teamwork is essential but often falters.
Our transdisciplinary research leverages insights from psychology, physiology, and computational sciences to analyze coordination dynamics in team interaction signals (e.g., heart rate, skin conductance, speech, motion) and identify (early warning signs of) team coordination breakdowns. We develop and test methods to convert these measurements into real-time feedback to help teams sustain effective team performance.
With studies in serious gaming and medical emergency simulation settings, we aim to generate actionable insights for future wearable applications, ultimately enhancing team functioning and adaptive performance in crisis conditions.
In this presentation, Josette will highlight the critical role of team coordination during crises, and Travis will detail the AI methodologies used to assess and potentially enhance these team dynamics.
Travis Wiltshire (duo talk)
Assistant Professor in the Department of Cognitive Science and Artificial Intelligence, Tilburg University
An AI-based feedback system for team support during crisis events
Wearable technology offers a groundbreaking opportunity to provide teams with real-time feedback to enhance their effectiveness in high-stakes crisis environments, such as medical emergencies.
View profile on Tilburg University website
Abstract
While current wearable technology primarily targets individual users, advancements in AI are unveiling new potentials for capturing and supporting team dynamics in circumstances where teamwork is essential but often falters.
Our transdisciplinary research leverages insights from psychology, physiology, and computational sciences to analyze coordination dynamics in team interaction signals (e.g., heart rate, skin conductance, speech, motion) and identify (early warning signs of) team coordination breakdowns. We develop and test methods to convert these measurements into real-time feedback to help teams sustain effective team performance.
With studies in serious gaming and medical emergency simulation settings, we aim to generate actionable insights for future wearable applications, ultimately enhancing team functioning and adaptive performance in crisis conditions.
In this presentation, Josette will highlight the critical role of team coordination during crises, and Travis will detail the AI methodologies used to assess and potentially enhance these team dynamics.
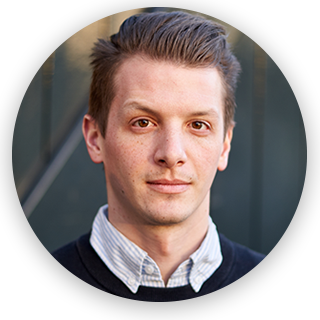
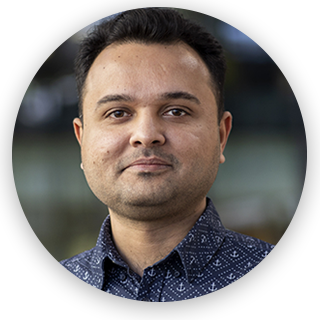
Aaqib Saeed (duo talk)
Decentralized AI for Sensing and Health
The ubiquity of interconnected systems has given rise to a world enriched with omnipresent computing, where computing is so ingrained in our daily lives that we often fail to realize our interactions with these platforms.
View profile Aaqib on TU/e website
Abstract
This proliferation of devices, embedded with sophisticated sensors, generates data at an unprecedented scale, presenting both opportunities and challenges for artificial intelligence (AI).
Decentralized AI is emerging as a core solution to effectively harness the power of distributed data and computing resources, enabling the development of data-driven predictive models that form the foundation of next-generation of embedded intelligence.
This talk will focus on our recent efforts in addressing the challenges associated with efficiently utilizing decentralized data in a scalable manner, particularly in the context of healthcare, where decentralized AI holds immense potential for revolutionizing patient care and improving health outcomes.
Hareld Kemps (duo talk)
Decentralized AI for Sensing and Health
The ubiquity of interconnected systems has given rise to a world enriched with omnipresent computing, where computing is so ingrained in our daily lives that we often fail to realize our interactions with these platforms.
View profile Maxima MC website
Abstract
This proliferation of devices, embedded with sophisticated sensors, generates data at an unprecedented scale, presenting both opportunities and challenges for artificial intelligence (AI).
Decentralized AI is emerging as a core solution to effectively harness the power of distributed data and computing resources, enabling the development of data-driven predictive models that form the foundation of next-generation of embedded intelligence.
This talk will focus on our recent efforts in addressing the challenges associated with efficiently utilizing decentralized data in a scalable manner, particularly in the context of healthcare, where decentralized AI holds immense potential for revolutionizing patient care and improving health outcomes.
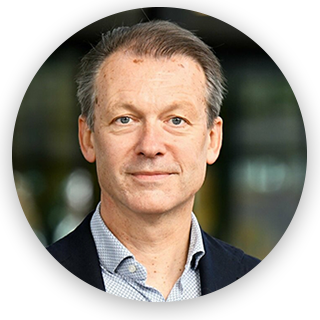
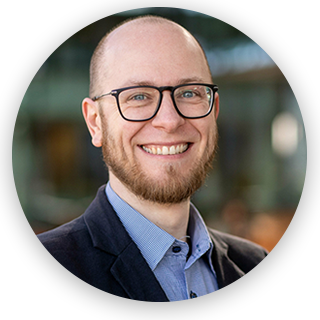
Jakub Tomczak
Associate professor TU/e
Generative AI Systems
Large Language Models (LLMs) have revolutionized AI systems by enabling communication with machines using natural language. Recent developments in Generative AI (GenAI) like Vision-Language Models (GPT-4V) and Gemini have shown great promise in using LLMs as multimodal systems.
View profile on LinkedIn
Abstract
This new research line results in building Generative AI systems, GenAISys for short, that are capable of multimodal processing and content creation, as well as decision-making. GenAISys use natural language as a communication means and modality encoders as I/O interfaces for processing various data sources.
They are also equipped with databases and external specialized tools, communicating with the system through a module for information retrieval and storage.
This presentation aims to explore and state new research directions in Generative AI Systems, including how to design GenAISys (compositionality, reliability, verifiability), build and train them, and what can be learned from the system-based perspective. Cross-disciplinary approaches are needed to answer open questions about the inner workings of GenAI systems.
Elena Torta
Collaborative robots for real-world applications: experiences from the EAISI Impuls research projects AMBER and TOWR
How can we increase the level of autonomy of collaborative robots? How can we leverage prior knowledge and machine learning to improve the performance of robotic navigation systems? In this talk we are going to explore these questions and more by looking at recent research results from the EAISI Impuls projects AMBER and TOWR
View profile on LinkedIn
Abstract
In this presentation we discuss recent results of the EAISI Impuls research projects AMBER and TOWR; a joint TU/e collaboration with Philips (AMBER) and Lely and Vanderlande (TOWR). In the talk we will show fundamental control and world modelling techniques and discus their usage in the development of complex navigation strategies for robotic solutions deployed in surgical and agricultural settings.
Emphasis will be given to optimization-based control methods (i.e., MPC) in conjunction with the usage of prior knowledge in the form of CAD or BIM models to provide input to the optimization problem. We will also discuss how machine learning and digital twins can provide helpful tools for the design and the automatic tuning of the navigation system.
Througout the presentation we will show how similar techniques can be applied to robots deployed in very different contexts, namely operating rooms and agricultural settings.
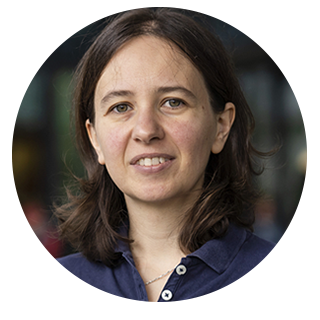
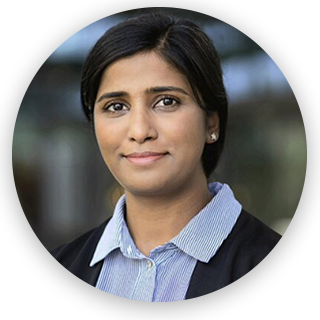
Zaharah Bukhsh (duo talk)
Assistant professor at Eindhoven University of Technology
Decision-making with deep reinforcement learning
Deep learning has revolutionized data-driven models, enabling the mastery of complex tasks by learning from vast amounts of (labeled) data.
View profile on TU/e website
Abstract
Deep reinforcement learning (RL) takes this progress a step further by enabling decision-making under uncertainty, where the goal is not only to perceive and classify but also to take actions and consider their long-term consequences.
In this talk, we will present real-world applications of deep RL in diverse domains, from maintenance planning of underground utilities and quay walls to the collaborative order picking problem in Vanderlande warehouses. We will show how deep RL empower intelligent decision-making in complex and dynamic environments.
The talk will conclude by providing valuable lessons learned from industry expert, shedding light on the challenges and opportunities that arise when applying deep RL in real-world settings.
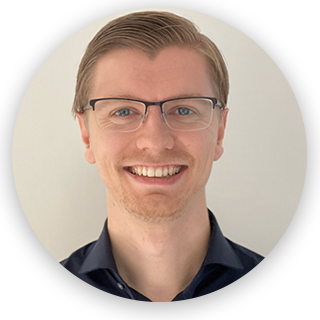
Kasper Hendriks (duo talk)
Innovation engineer at Vanderlande
Decision-making with deep reinforcement learning
Deep learning has revolutionized data-driven models, enabling the mastery of complex tasks by learning from vast amounts of (labeled) data.
View profile on LinkedIn
Abstract
Deep reinforcement learning (RL) takes this progress a step further by enabling decision-making under uncertainty, where the goal is not only to perceive and classify but also to take actions and consider their long-term consequences.
In this talk, we will present real-world applications of deep RL in diverse domains, from maintenance planning of underground utilities and quay walls to the collaborative order picking problem in Vanderlande warehouses. We will show how deep RL empower intelligent decision-making in complex and dynamic environments.
The talk will conclude by providing valuable lessons learned from industry expert, shedding light on the challenges and opportunities that arise when applying deep RL in real-world settings.
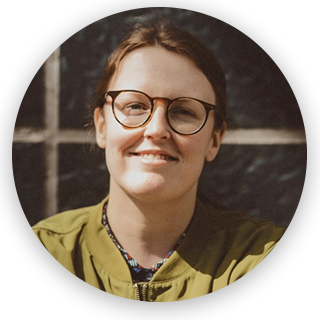
Patricia Kahr
PhD Candidate in the Human-Technology Interaction group.
Performance is Not Everything! Learning What Promotes Trustworthy Human-AI Collaborations. Insights from a Case Study in Logistics Planning
An important area of research on human-AI interaction focuses on promoting trust and reliance in AI decision support systems.
View profile on TU/e website
Abstract
Research in this area is thriving, and it is evident that trust and reliance in AI systems are often not well-calibrated for users: experts frequently find it challenging to depend on the advice from such systems or may outright refuse to use their support for decision-making.
To gain a deeper understanding of real-life collaborations, we conducted semi-structured in-depth interviews with logistics planning experts to explore their experiences with their automated planning system.
Our study sheds light on the overall trust dynamics in this area and the conditions under which experts rely on or deviate from AI recommendations. We highlight the importance of incorporating human needs and special circumstances into AI systems to foster more effective and trustworthy collaboration between humans and AI.
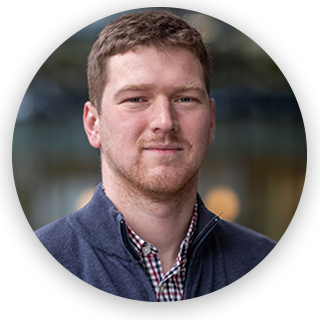
Andrii Kompanets
PhD Candidate at the Steel Structures group at TU/e
AI-aided visual inspection of steel bridges
Automating the current bridge visual inspection practices using drones and image processing techniques is a prominent way to make these inspections more effective, robust, and less expensive.
View profile on TU/e website
Abstract
In our work, we investigate the development of a novel deep-learning method for the detection of fatigue cracks in high-resolution images of steel bridges.
First, we developed a semi-automatic tool based on a geometric tracking algorithm, which allows a fast and accurate pixels-wise annotation of cracks in images. We used the developed tool to generate a challenging benchmark dataset. Further, we conducted series of experiments on this dataset, aiming at the development of a robust, fully-automatic deep-learning method for crack segmentation.
Based on these experiments, multiple discoveries were made and novel solutions were proposed, which helped to significantly increase reliability of crack segmentation system.
To prove the concept of automatic inspection of bridges, we also developed a method to compare our automatic crack segmentation system with the performances of human inspectors.
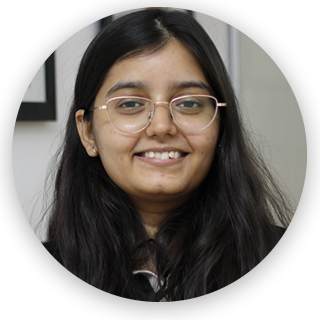
Apoorva Singh
Predictive modeling of large-scale pedestrian dynamics using AI
The current research work, supported by the EAISI EMDAIR project “AICrowd”, aims at quantitatively modelling the dynamics of pedestrian crowds, using tools from AI and System Identification.
View profile on TU/e website
Abstract
It's part of a decade-long endeavor towards establishing a physical understanding and predictive modeling capabilities of crowd flows: an effort at the interface of physics, AI, and engineering.
This understanding is crucial for effective crowd management and designing safe and comfortable urban infrastructure.
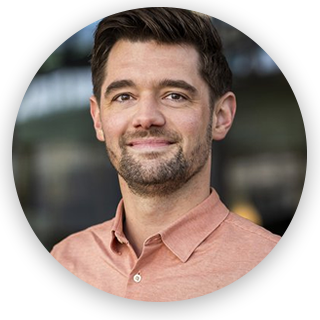
Fons van der Sommen
Associate Professor Video Coding & Architectures, TU/e
Robust, self-critical AI for oncology
While the capabilities of modern AI systems keep surpassing expectations for a wide range of applications, their development has mostly driven by their accuracy on standardized data sets.
View profile on TU/e website
Abstract
This empirical approach to model development introduces blind spots towards their application, as evidenced by an increasing number of reports on unstable model behaviour, under minor changes in the unput data.
For healthcare applications, these observations are especially concerning, as unreliable AI output may lead to incorrect diagnoses and treatment.
In this talk, dr. van der Sommen will elaborate on the current lack of model robustness for oncology applications and will discuss methods that could help mitigate this problem.
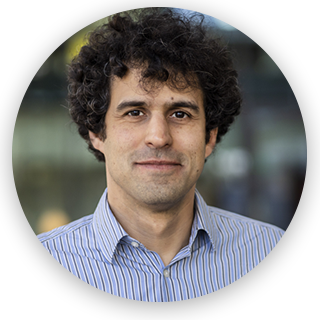
Carlos Zednik
Assistant Professor, TU/e
Cognitive models to understand knowledge-representation in large language models
Harnessing the power of large language models while retaining control over their behavior is one of the central challenges of contemporary artificial intelligence.
View profile on TU/e website
Abstract
One way to meet this challenge is to identify and characterize the knowledge these models possess, or the beliefs that drive their behavior.
In this talk, I present an explanatory strategy that combines methodological principles from cognitive science and explainable AI to characterize the knowledge or beliefs that are represented in state-of-the-art transformer architectures.
This strategy centers on cognitive models, which provide not only predictive power over a particular system's behavior, but which also support systematic and targeted interventions on that behavior.
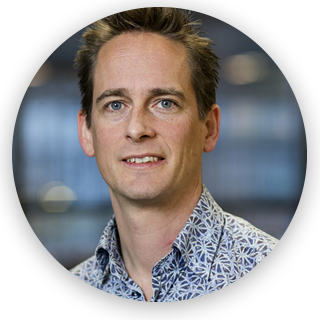
Remco Duits
Head of the Geometric Learning and Differential Geometry group, Cluster: CASA. Department: Mathematics and Computer Science. EAISI, TU/e.
New Geometric Learning for Medical and Industrial Image Analysis
Our geometrically interpretable networks achieve better classification results in image processing with both less training data and less network complexity.
View profile on TU/e website
Abstract
We show that our geometric image processing is more accurate for various medical and industrial image analysis problems, e.g.: 1) segmentation and tracking of complex vasculature, 2) wall-shear stress estimation of vasculature, 3) segmentation of chips, and 4) crack detection in steel bridges.
To get to state-of-the-art solutions for practical medical and industrial image analysis problems, we develop PDE-based Group Convolutional Neural Networks (PDE-G-CNNs).
In PDE-G-CNNs a network layer is a set of solvers of PDEs (Partial Differential Equations). The PDEs are defined on the space of positions and orientations and provide a geometric design of the roto-translation equivariant neural network.
The network consists of morphological convolutions with kernels solving nonlinear PDEs (HJB equations for max-pooling over Riemannian balls), and linear convolutions solving linear PDEs (convection, fractional diffusion). Common mystifying (ReLU) nonlinearities are now obsolete and excluded.
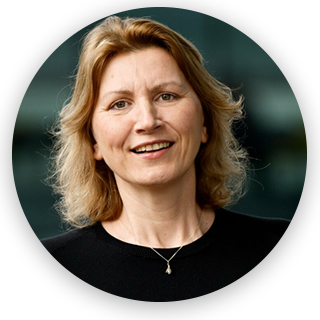
Josette Gevers (duo talk)
Full Professor TU/e
An AI-based feedback system for team support during crisis events
Wearable technology offers a groundbreaking opportunity to provide teams with real-time feedback to enhance their effectiveness in high-stakes crisis environments, such as medical emergencies.
View profile on TU/e website
Abstract
While current wearable technology primarily targets individual users, advancements in AI are unveiling new potentials for capturing and supporting team dynamics in circumstances where teamwork is essential but often falters.
Our transdisciplinary research leverages insights from psychology, physiology, and computational sciences to analyze coordination dynamics in team interaction signals (e.g., heart rate, skin conductance, speech, motion) and identify (early warning signs of) team coordination breakdowns. We develop and test methods to convert these measurements into real-time feedback to help teams sustain effective team performance.
With studies in serious gaming and medical emergency simulation settings, we aim to generate actionable insights for future wearable applications, ultimately enhancing team functioning and adaptive performance in crisis conditions.
In this presentation, Josette will highlight the critical role of team coordination during crises, and Travis will detail the AI methodologies used to assess and potentially enhance these team dynamics.
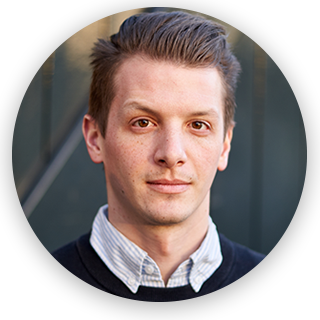
Travis Wiltshire (duo talk)
Assistant Professor in the Department of Cognitive Science and Artificial Intelligence, Tilburg University
An AI-based feedback system for team support during crisis events
Wearable technology offers a groundbreaking opportunity to provide teams with real-time feedback to enhance their effectiveness in high-stakes crisis environments, such as medical emergencies.
View profile on Tilburg University website
Abstract
While current wearable technology primarily targets individual users, advancements in AI are unveiling new potentials for capturing and supporting team dynamics in circumstances where teamwork is essential but often falters.
Our transdisciplinary research leverages insights from psychology, physiology, and computational sciences to analyze coordination dynamics in team interaction signals (e.g., heart rate, skin conductance, speech, motion) and identify (early warning signs of) team coordination breakdowns. We develop and test methods to convert these measurements into real-time feedback to help teams sustain effective team performance.
With studies in serious gaming and medical emergency simulation settings, we aim to generate actionable insights for future wearable applications, ultimately enhancing team functioning and adaptive performance in crisis conditions.
In this presentation, Josette will highlight the critical role of team coordination during crises, and Travis will detail the AI methodologies used to assess and potentially enhance these team dynamics.
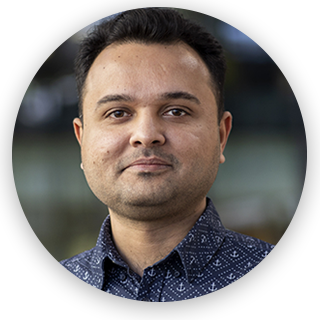
Aaqib Saeed (duo talk)
Decentralized AI for Sensing and Health
The ubiquity of interconnected systems has given rise to a world enriched with omnipresent computing, where computing is so ingrained in our daily lives that we often fail to realize our interactions with these platforms.
View profile Aaqib on TU/e website
Abstract
This proliferation of devices, embedded with sophisticated sensors, generates data at an unprecedented scale, presenting both opportunities and challenges for artificial intelligence (AI).
Decentralized AI is emerging as a core solution to effectively harness the power of distributed data and computing resources, enabling the development of data-driven predictive models that form the foundation of next-generation of embedded intelligence.
This talk will focus on our recent efforts in addressing the challenges associated with efficiently utilizing decentralized data in a scalable manner, particularly in the context of healthcare, where decentralized AI holds immense potential for revolutionizing patient care and improving health outcomes.
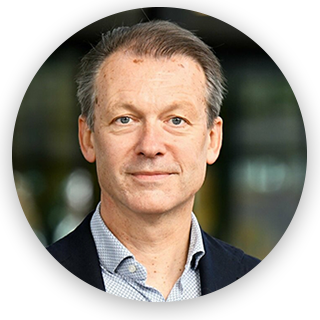
Hareld Kemps (duo talk)
Decentralized AI for Sensing and Health
The ubiquity of interconnected systems has given rise to a world enriched with omnipresent computing, where computing is so ingrained in our daily lives that we often fail to realize our interactions with these platforms.
View profile Maxima MC website
Abstract
This proliferation of devices, embedded with sophisticated sensors, generates data at an unprecedented scale, presenting both opportunities and challenges for artificial intelligence (AI).
Decentralized AI is emerging as a core solution to effectively harness the power of distributed data and computing resources, enabling the development of data-driven predictive models that form the foundation of next-generation of embedded intelligence.
This talk will focus on our recent efforts in addressing the challenges associated with efficiently utilizing decentralized data in a scalable manner, particularly in the context of healthcare, where decentralized AI holds immense potential for revolutionizing patient care and improving health outcomes.
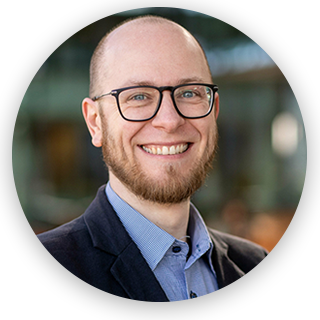
Jakub Tomczak
Associate professor TU/e
Generative AI Systems
Jakub M. Tomczak is an Associate Professor and the Head of the Generative AI group at the Eindhoven University of Technology. He serves as a Program Chair of NeurIPS 2024. He is the founder of Amsterdam AI Solutions. His research interests are Generative AI, Deep Learning and Probabilistic Modeling.
View profile on LinkedIn
Abstract
Large Language Models (LLMs) have revolutionized AI systems by enabling communication with machines using natural language. Recent developments in Generative AI (GenAI) like Vision-Language Models (GPT-4V) and Gemini have shown great promise in using LLMs as multimodal systems.
This new research line results in building Generative AI systems, GenAISys for short, that are capable of multimodal processing and content creation, as well as decision-making. GenAISys use natural language as a communication means and modality encoders as I/O interfaces for processing various data sources.
They are also equipped with databases and external specialized tools, communicating with the system through a module for information retrieval and storage.
This presentation aims to explore and state new research directions in Generative AI Systems, including how to design GenAISys (compositionality, reliability, verifiability), build and train them, and what can be learned from the system-based perspective. Cross-disciplinary approaches are needed to answer open questions about the inner workings of GenAI systems.
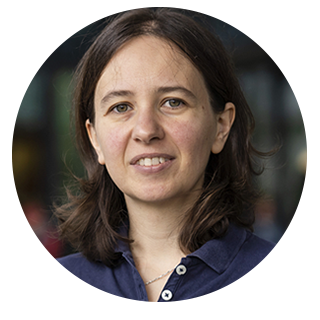
Elena Torta
Collaborative robots for real-world applications: experiences from the EAISI Impuls research projects AMBER and TOWR
How can we increase the level of autonomy of collaborative robots? How can we leverage prior knowledge and machine learning to improve the performance of robotic navigation systems? In this talk we are going to explore these questions and more by looking at recent research results from the EAISI Impuls projects AMBER and TOWR
View profile on LinkedIn
Abstract
In this presentation we discuss recent results of the EAISI Impuls research projects AMBER and TOWR; a joint TU/e collaboration with Philips (AMBER) and Lely and Vanderlande (TOWR). In the talk we will show fundamental control and world modelling techniques and discus their usage in the development of complex navigation strategies for robotic solutions deployed in surgical and agricultural settings.
Emphasis will be given to optimization-based control methods (i.e., MPC) in conjunction with the usage of prior knowledge in the form of CAD or BIM models to provide input to the optimization problem. We will also discuss how machine learning and digital twins can provide helpful tools for the design and the automatic tuning of the navigation system.
Througout the presentation we will show how similar techniques can be applied to robots deployed in very different contexts, namely operating rooms and agricultural settings.
MORNING PROGRAM
09:00 |
Walk in with tea/coffee |
AFTERNOON PROGRAM
13:00 |
Lunch break & Expo |
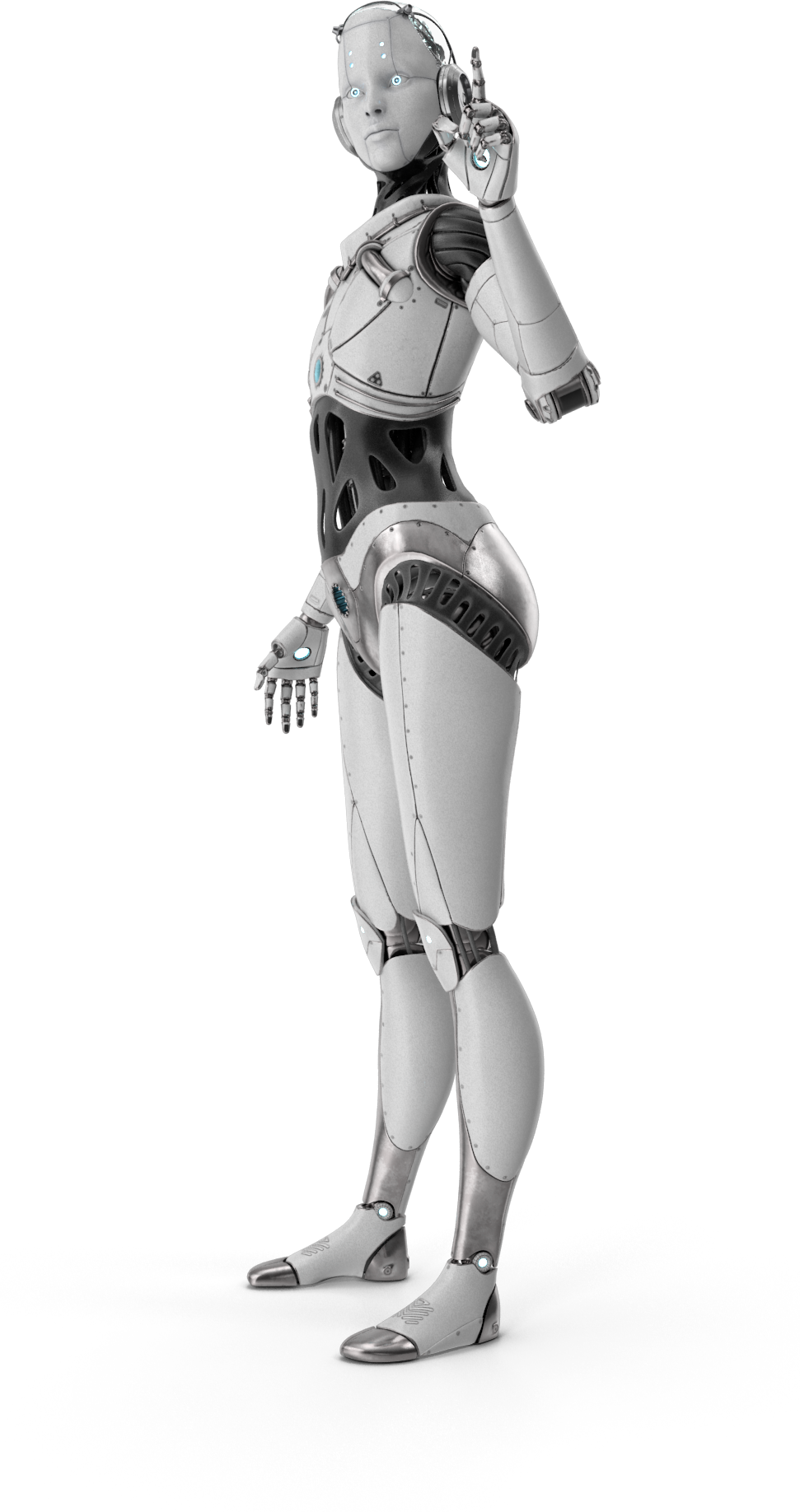
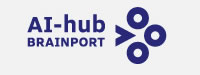
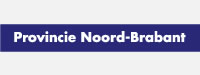
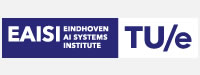